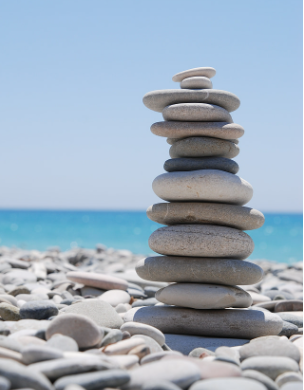
Educational Neuroscience Comprehensive Examinations
- Teacher: Mohamed Fahim
- Teacher: Claudine Habak
- Teacher: Mohamed Lamine Seghier
- Teacher: Kyongje Sung
Skill Level: Beginner
- Teacher: Michael Melkonian
Skill Level: Beginner
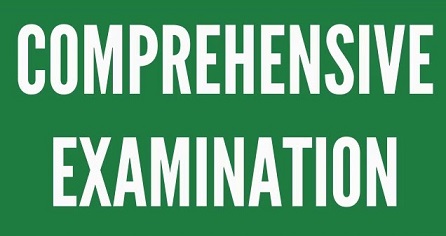
- Teacher: Shaljan Areepattamannil
Skill Level: Beginner
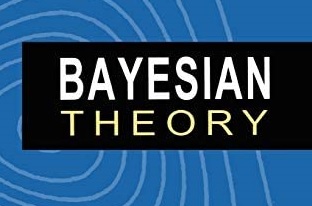
This course provides a thorough and comprehensive overview of Bayesian psychometric modelling. It presents a unified Bayesian approach across traditionally separate families of psychometric models. It shows that Bayesian techniques, as alternatives to conventional approaches, offer distinct and profound advantages in achieving many goals of psychometrics. This course explains both how to perform psychometrics using Bayesian methods and why many of the activities in psychometrics align with Bayesian thinking. The course starts with simple models and then builds from them to develop more complicated models. Throughout the course, procedures will be illustrated using examples primarily drawn from educational assessments. The course will use Mplus/Stata software packages.
- Teacher: Shaljan Areepattamannil
Skill Level: Beginner
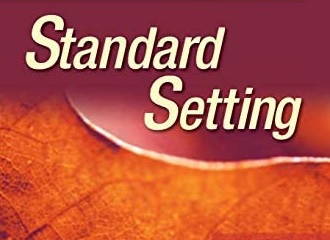
This course provides an in-depth treatment of modern standard setting methods. The course presents the state-of-the-art in contemporary thinking about the science and practice of setting performance standards. It reflects critically on what the future of standard setting theory and practice can, should, and might accomplish. The course provides the most current and forward-looking review of the major methods used for setting standards today, and methods that are likely to assume greater prominence in the years to come. Beyond merely describing each method, it provides a summary of research on the method and a critical appraisal of strengths, weaknesses, limitations, and recommendations for future research.
- Teacher: Shaljan Areepattamannil
Skill Level: Beginner
This course lays out the conceptual and statistical foundations of univariate and multivariate theory. It also provides a detailed coverage of advanced topics in univariate and multivariate generalizability theory. Beginning with single-facet designs, the course progresses through multi-facet universes and G-study designs and random effects D-study designs. Both crossed and nested designs are presented. Advanced topics covered include D-study procedures for situations involving restricted universes of generalization and sampling from finite universes; effects of hidden facets, stratified objects of measurement, cautions regarding reliability of groups means, conditional standard errors of measurement, universe score estimation, and comparison of generalizability theory with other measurement theories. Lab sessions will have students develop data analytic skills using the generalizability theory computer programs generalizability, such as GENOVA, urGENOVA, and mGENOVA.
- Teacher: Rehab Al Hekmani
- Teacher: Dean Cairns
Skill Level: Beginner